SITUATION
A global pharmaceutical leader was preparing to launch multiple large-scale immunology trials. Despite robust planning models, they struggled with operational bottlenecks during execution — particularly around resource overload, protocol overlap, and last-minute firefighting that slowed timelines and increased costs.
CHALLENGE
Traditional predictive models, built on static historical data, failed to account for real-time disruptions from CRA workload spikes to protocol complexities and regulatory shifts. Overreliance on these models led to planning fallacies, unbalanced trial execution, and delayed milestones.
APPROACH
Mu Sigma partnered with the client to deploy a Digital Twin cockpit, a simulation engine combining complexity science, agent-based modeling, and real-world trial data.
- Simulated Environment:Created virtual representations of over 20 protocols, 5,000 sites, and 700 CRA portfolios
- Agent Modeling:Represented key trial agents such as sites, patients, protocols, CRAs, and reviewers with dynamic interactions
- Disruption Testing:Ran “what-if” scenarios to uncover hidden risks like capacity bottlenecks and delayed site activations
- Diagnostic + Validation Modules: Enabled real-time cross-checking of assumptions, allowing users to refine strategy proactively
- Outcome-Oriented Execution: Powered operational decisions using an OODA (Observe–Orient–Decide–Act) loop approach
IMPACT
- Eliminated CRA bottlenecks during critical trial weeks by proactively reallocating resources
- Reduced operational firefighting through real-time strategy adjustments and disruption forecasting
- Empowered leadership with a live portfolio cockpit to track evolution and drive evidence-based decisions
- Created a scalable framework that can be reused across future trials to improve readiness and reduce risk
Business Impact
-
0
CRA Bottlenecks
-
Disruption Forecasting
Let’s move from data to decisions together. Talk to us.
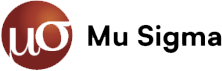
The firm's name is derived from the statistical terms "Mu" and "Sigma," which symbolize a
probability distribution's mean and standard deviation, respectively.