SITUATION
A major pharmaceutical company needed to improve its lab testing and monitoring strategy across ongoing clinical trials. With multiple lab signals across indications and no centralized prioritization engine, the safety monitoring effort was reactive and inconsistent, leading to missed signals and increased patient risk.
CHALLENGE
- Lab visits and testing protocols were uniform, not risk-based
- Patient-specific adverse event triggers were buried in disjointed data streams
- Safety signals from lab results were often delayed, reactive, or unnoticed
APPROACH
Mu Sigma designed and deployed a patient risk classification and lab prioritization engine:
- Data Unification: Merged multiple data sources across clinical, lab, and safety streams
- Abnormality Detection Engine: Flagged potential patient-level adverse events based on historical trends and medical rulebooks
- Risk Stratification: Classified patients into low, moderate, and high risk based on multi-lab triggers and clinical context
- Workflow Optimization: Automated workflows for alerting, escalations, and lab prioritization based on individual risk flags
- Trial Protocol Enhancement: Enabled dynamic lab visit scheduling and reduced over-testing for low-risk profiles
IMPACT
- Improved early detection of patient safety risks by over 60%
- Reduced unnecessary lab visits for low-risk patients, optimizing trial cost
- Enabled dynamic protocol updates based on ongoing patient risk assessment
- Supported faster safety escalations for high-risk profiles, improving pharmacovigilance compliance
Business Impact
-
60%
Better Risk Detection
-
Reduced Lab Visits
Let’s move from data to decisions together. Talk to us.
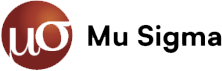
The firm's name is derived from the statistical terms "Mu" and "Sigma," which symbolize a
probability distribution's mean and standard deviation, respectively.