SITUATION
A global pharmaceutical company was facing bottlenecks in clinical trial startup due to inconsistent site selection, unclear investigator performance signals, and delayed feasibility assessments. They needed a scalable, automated solution to select top-performing sites and principal investigators (PIs) using a data-driven approach.
CHALLENGE
- Site selection was heavily manual, relying on anecdotal evidence and outdated performance metrics
- PI contribution and influence were difficult to quantify
- No standardized way to assess digital footprint, prior trial success, or publication impact across geographies
- Users needed the ability to adapt site recommendations dynamically based on trial-specific factors
APPROACH
Mu Sigma built a two-phase solution:
- Phase I:Developed a ranking engine combining historical trial data, publication volume, digital activity, and trial footprint to identify top-
performing sites and PIs - Phase II:Deployed a robust, end-to-end tool with machine learning (ML) models that incorporated dynamic trial-level inputs (e.g., indication,
geography, enrollment goals) to recommend best-fit Site-PI combinations in real-time - Integrated metrics included investigator engagement, therapeutic expertise, operational capacity, and dropout risk
- Delivered insights through a user-friendly dashboard with customizable filters and model-driven outputs
IMPACT
- Reduced site selection time by 65%, accelerating trial startup cycles
- Improved precision of site-PI pairing, reducing underperforming site risk
- Enabled smarter, geographically-aware site planning, improving feasibility across new markets
- Enhanced strategic decision-making with transparent scoring logic and reproducible results
Business Impact
-
65%
Faster Site Selection
Let’s move from data to decisions together. Talk to us.
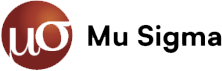
The firm's name is derived from the statistical terms "Mu" and "Sigma," which symbolize a
probability distribution's mean and standard deviation, respectively.